Light
Brands engage with consumers through a lot of channels. But the data they gather from these disparate sources often remains scattered, siloed and fragmented across platforms. This makes it difficult to effectively define and optimize customer journeys.
Customer data platforms (CDPs) have emerged to fix this fragmentation. These systems are specifically tailored to gather and integrate multi-channel data to build a unified customer profile.
With so much data available through so many systems and channels, CDPs are becoming a necessity. The CDP market’s value is predicted to expand from around $3.77 billion to nearly $13 billion by 2030 – and for good reason. Businesses and marketers are realizing the increasing need of data management, personalized marketing strategies and unified customer experiences.
The Need for a Unified Customer Experience
Consistency across touchpoints
Modern consumers interact with brands across websites, mobile apps, social media, physical stores, customer service and many other channels. A unified customer experience ensures that regardless of the touchpoint, customers meet the same tone, messaging and functionality. This continuity builds trust and encourages customers to engage more with the brand.
Enhanced customer satisfaction
Fragmented experiences often frustrate customers, especially when they have to repeat their concerns or re-enter their data at different stages of interaction. A unified experience removes this friction. For instance, when a customer starts a support chat on a website and then calls customer service, agents should have access to the existing chat history. This continuity not only saves the customer time but also makes them feel valued, which strengthens brand loyalty and overall satisfaction.
Streamlined communication
Customers today expect personalized and relevant messaging, but disjointed systems make it hard to deliver these experiences. Centralizing customer data from various platforms allows you to send consistent, well-timed messages that are appropriate to the customer’s journey. For example, rather than bombarding a customer with promotions for items they’ve already bought, you can recommend complementary products. This targeted communication increases engagement and reduces the risk of alienating customers with irrelevant or excessive messaging.
Stronger brand identity
A consistent and unified experience amplifies your brand’s identity. Whether a customer interacts with your brand through social media, a support call or an in-store visit, they should experience the same core values and service standards. A unified approach ensures that every touchpoint reinforces your brand promise, making the customer feel connected to your mission and values.
Improved operational efficiency
Disconnected systems and workflows are inefficient, and can cause issues like redundant data entry, delays in responding to customer queries or misaligned strategies between teams. By integrating systems, you can streamline processes, reduce manual errors and ensure that every team, whether marketing, sales or support, has access to the same customer information.
Understanding CDPs and How They Impact Customer Experiences
Several emerging MarTech tools aim to create a comprehensive customer profile. These tools include social media listening tools, CRM software, the voice of the customer programs, web analytics and marketing automation. However, all of these continuously generate new data that must be integrated, cleaned and made accessible. A CDP can consolidate this dispersed data into a single source.
CDPs allows you to aggregate customer data from all touchpoints to create a unified, 360-degree view of your customers. As a result, marketing campaigns become data-driven and customer-centric. This approach ensures a seamless experience throughout the entire customer journey.
By unifying data, CDPs also help you build customer experience (CX) programs more quickly and at a lower cost. This unified data is crucial for understanding and engaging with customers. It enables informed decision-making, enhances customer experiences and improves conversion rates.
Think of a CDP as a data hub, rather than just a database, repository or CRM system. It exposes, aggregates, formats and directs customer data from multiple sources and channels toward specific tasks
CDPs can help you accomplish a lot, including the following:
-
Gather data on user actions from your website, app or mobile browser;
-
Store personal details such as names, addresses, contact information and birthdays to enable machine-learning-powered predictions;
-
Maintain records of purchases, returns and other interactions on e-commerce platforms;
-
Gauge engagement metrics, reach, impressions and other campaign data; and
-
Collect information from customer service interactions and live chats, NPS scores and other data from CRM systems.
Just remember, before collecting and using consumer data, it’s essential to obtain consent. This is essential for compliance with regulations like the EU’s GDPR and California’s data privacy laws; it’s also vital for building trust.
The Benefits of Customer Data Platforms
Track customer journeys
By analyzing customer data from various touchpoints, CDPs enhance personalization and optimize customer journeys at every stage.
Personalize experiences
CDPs provide marketers with accurate and detailed customer profiles by integrating data from CRM systems, emails and websites. Personalized emails and texts resonate with customers on an individual level, capturing their attention and driving conversions. In fact, 75% of consumers say personalized communications are key to choosing a brand and making purchases.
Get real-time results
The amount of time it takes to prepare data, identify trends and create customized campaigns can lead to missed opportunities. CDPs offer real-time personalization, helping you nurture customer relationships and drive sales at critical moments. They deliver relevant content exactly when it’s needed.
Salesforce Data Cloud is a perfect example of real-time data integration; it serves as a robust platform that drives a 360-degree customer view. It allows you to transform data into personalized customer interactions, adapting instantly to changes in customer behavior and needs across sales, service and marketing channels.
With Salesforce’s Data Cloud, you can seamlessly connect real-time data from both Salesforce and external sources. This capability enables you to create smarter customer segments up to 30 times faster – ensuring the right message is delivered at the right moment across multiple channels.
According to Salesforce research, 77% of customers expect an immediate response when they reach out to a company. The report also highlights that customers appreciate consistent and unified experiences across channels, devices, and departments.
“A unified experience means that customers are treated consistently across all channels and that each experience is tailored to the customer’s personal situation.” – David Raab, founder of the CDP Institute
While training can help your team interact with customers consistently across channels, access to customer data is essential for creating truly personalized experiences. Achieving consistency requires synchronizing all customer-facing interactions with a CDP and adhering to unified guidelines.
What Makes CDPs Different from CRMs and Other Data Management Platforms?
As technology has evolved and more channels and kinds of data have been introduced, new tools for managing it all have emerged.
Online customer relationship management (CRM) software appeared in the 1990s, allowing businesses to record interactions with both current and potential customers in one place. While CRMs helped analyze data to improve retention and sales, they were limited to registered clients and predefined data sets.
In the 2000s, Data Management Platforms (DMPs) transformed data handling. They were able to facilitate the planning and execution of media campaigns using second and third-party data. DMPs could also segment anonymous user IDs.
CDPs were introduced in 2016 to enhance customer experience and support omnichannel marketing. This innovation arose from the need to effectively use the vast amounts of data available to marketers.
The table below summarizes the distinctions among CDPs, DMPs and CRMs to help you choose the right solution for your business.
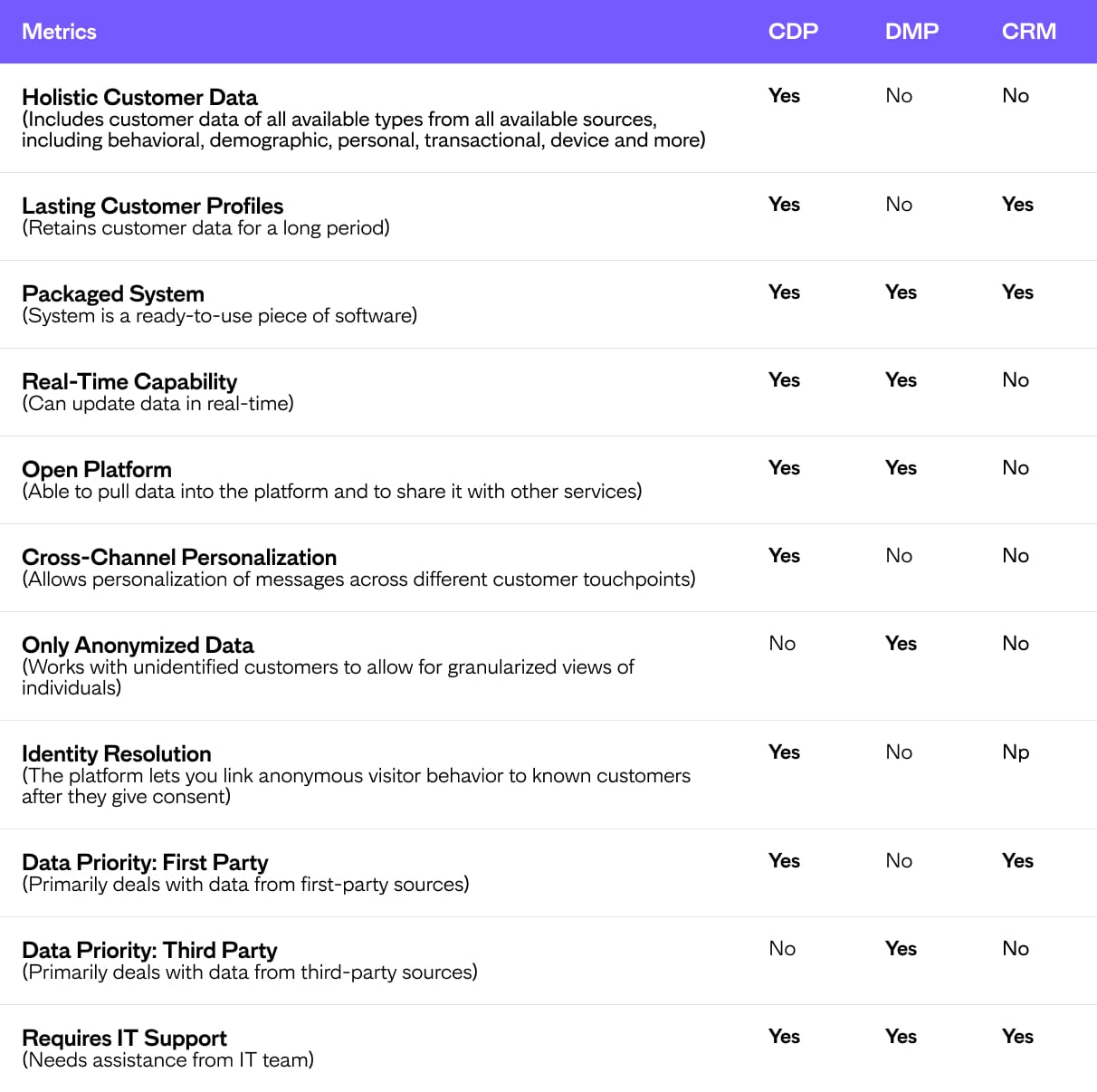
Implement and Manage Your CDP with Material
As MarTech continues to evolve across channels and categories, the volume of structured and unstructured data grows. The rapid rise of customer data platforms (CDPs) underscores the need to make this data actionable.
Making the most of your data, however, requires more than a CDP. It requires expertise in implementing and optimizing your system, and developing a strategy based on the insights it delivers.
This is where Material can be a powerful partner. We have deep expertise in marketing technology, behavioral science and creating personalized experiences. We can help you strategically implement, manage and fine-tune your CDP to increase marketing efficiency, generate engagement and drive better business outcomes.
If you’re ready to enjoy the benefits of a customer data platform, reach out today and let’s start the conversation.